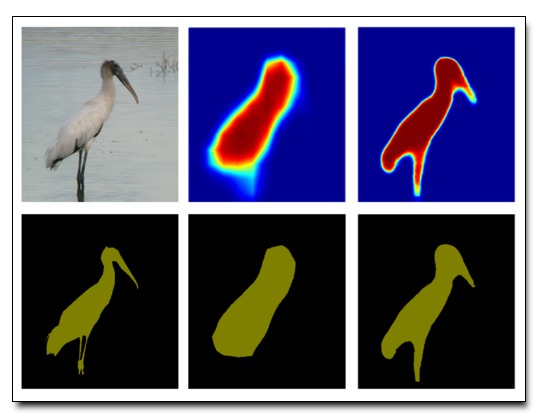
CNN architectures have terrific recognition performance
but rely on spatial pooling which makes it difficult to adapt
them to tasks that require dense, pixel-accurate labeling.
This paper makes two contributions: (1) We demonstrate that
while the apparent spatial resolution of convolutional feature
maps is low, the high-dimensional feature representation
contains significant sub-pixel localization information.
(2) We describe a multi-resolution reconstruction architecture
based on a Laplacian pyramid that uses skip connections
from higher resolution feature maps and multiplicative
gating to successively refine segment boundaries reconstructed
from lower-resolution maps. This approach yields
state-of-the-art semantic segmentation results on the PASCAL VOC
and Cityscapes segmentation benchmarks without resorting to
more complex random-field inference or instance detection
driven architectures.
Download: pdf
Text Reference
Golnaz Ghiasi and Charless Fowlkes.
Laplacian pyramid reconstruction and refinement for semantic segmentation.
In
ECCV. 2016.
BibTeX Reference
@inproceedings{GhiasiF_ECCV_2016,
AUTHOR = "Ghiasi, Golnaz and Fowlkes, Charless",
TITLE = "Laplacian Pyramid Reconstruction and Refinement for Semantic Segmentation",
BOOKTITLE = "ECCV",
YEAR = "2016",
tag = "grouping,object_recognision"
}