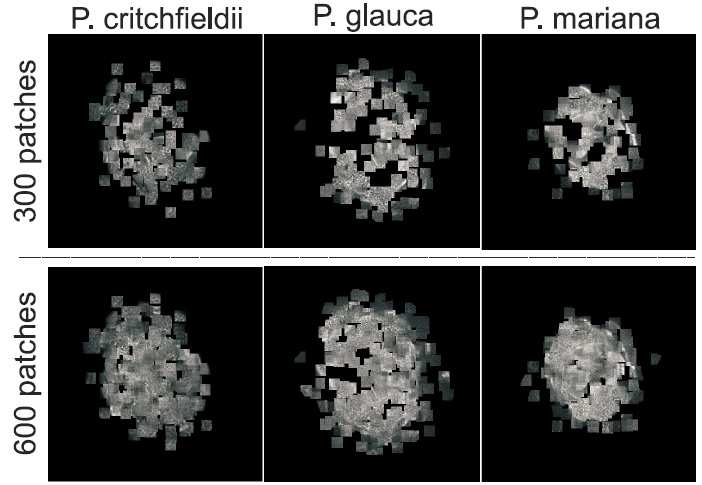
We propose a robust approach for performing automatic species-level recognition of fossil pollen grains in microscopy images that exploits both global shape and local texture characteristics in a patch-based matching methodology. We introduce a novel criteria for selecting meaningful and discriminative exemplar patches. We optimize this function during training using a greedy submodular function optimization framework that gives a near-optimal solution with bounded approximation error. We use these selected exemplars as a dictionary basis and propose a spatially-aware sparse coding method to match testing images for identification while maintaining global shape correspondence. To accelerate the coding process for fast matching, we introduce a relaxed form that uses spatially-aware soft-thresholding during coding. Finally, we carry out an experimental study that demonstrates the effectiveness and efficiency of our exemplar selection and classification mechanisms, achieving 86.13% accuracy on a difficult fine-grained species classification task distinguishing three types of fossil spruce pollen.
Download: pdf
Text Reference
Shu Kong, Surangi Punyasena, and Charless Fowlkes.
Spatially aware dictionary learning and coding for fossil pollen identification.
In
CVPR workshop CVMI. 2016.
BibTeX Reference
@inproceedings{KongPF_CVPRW_2016,
AUTHOR = "Kong, Shu and Punyasena, Surangi and Fowlkes, Charless",
TITLE = "Spatially Aware Dictionary Learning and Coding for Fossil Pollen Identification",
BOOKTITLE = "CVPR workshop CVMI",
YEAR = "2016",
tag = "grouping,object_recognision,biological_images"
}